HU2U Podcast: Encouraging Diversity in Data Analytics and What It Means To You
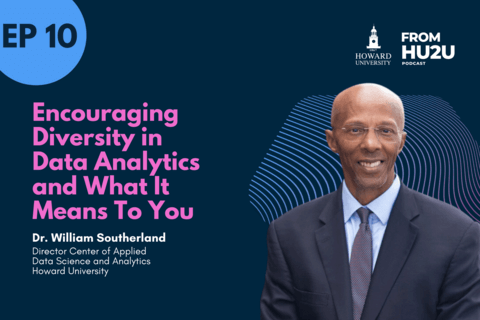
In This Episode
In the past five years, the field of data science skyrocketed in the United States from 1,700 jobs in 2016, to more than 10,000 jobs in 2021. But black scientists, scholars, and researchers make up only 3% of the professionals who interpret data and analytics.
Today, we sit down with Dr. William Southerland. He is a professor of biochemistry and molecular biology, principal investigator of the HU Research Centers for Minorities Institute's program.
Host: Frank Tramble, former VP of Communications at Howard University
Guest: Dr. William Southerland
Listen on all major podcast platforms
Episode Transcript
Encouraging Diversity in Data Analytics and What It Means To You feat. Dr. William Southerland
Publishing Date: Jan 29, 2024
[00:00:00] Frank: How you get a mortgage, what type of health insurance you qualify for, or what school your child gets into is based on how data statistics are interpreted. In the past five years, the field of data science skyrocketed in the United States, from 1,700 jobs in 2016 to more than 10,000 jobs in 2021. But black scientists, scholars, and researchers make up only 3% of the professionals who interpret data and analytics. Let's dig into it.
Welcome to HU2U, the podcast where we bring today's important topics and stories from Howard University right to you. I'm Frank Tramble, today's host, and I'm here with Dr. William Southerland, professor of biochemistry and molecular biology, and principal investigator of the HU Research Centers for Minorities Institutes Program, an interim director of the new Center of Applied Data Science and Analytics at Howard. Welcome to our podcast, Dr. Southerland. How you doing?
[00:01:00] William: Very well. Pleased to be here.
[00:01:02] Frank: All right, all right. Let's start off. Why is it important for diversity to be something inside of data and analytics?
[00:01:09] William: Well, people often say that the numbers speak for themselves why is it important to have diversity in the interpretation and the analysis. And it is true, the numbers do speak, but it's not necessarily accurate that they speak for themselves because when you say that numbers speak, what you're really saying is that numbers convey information.
And in order for the numbers to convey information, that information has to be extracted from those numbers via an analysis. And that's really an important aspect of data science. Let me give you an example. Suppose there's a financial services company and that company has two data scientists, and the role of those data scientists is to help the company make decisions on who's a good lending risk, the credit worthiness of their clients.
Those individuals will factor into the algorithm their own inherent biases, and their biases is really a reflection of their accumulated life experiences of what I call their experiential DNA. For example, one data scientist can say, "Well, I believe if you are a homeowner living in a certain neighborhood, then you are likely to be a good credit risk because of your ZIP code basically."
Another data scientist can look at the same data and say, "Well, there are reasons, there are historical reasons why some people may not live in that space or, or may not live in that ZIP code." So, when the first data scientist would analyze that, he or she would say, in their algorithm, they would give extra weight to the ZIP code, for example, and that would influence the recommendation.
Whereas the second data scientist, she would realize that the ZIP code a person is, is living at right now, there are some historical reasons why it's been more difficult for some people living at the ZIP code than others. And then as a result, she would give less weight to those criteria.
So, then you got two data scientists looking at the same data, really would make different recommendations to the financial services company. So, those numbers are speaking but they're saying different things based on the experiential DNA, of what I call it, of the data scientists.
[00:03:38] Frank: And that makes so much sense. I think numbers without context is, is the important part of data.
[00:03:42] William: Exactly, exactly.
[00:03:43] Frank: You know, there, there's a stat once upon a time in the marketing world when you read e-mails, you can look at open rates, right?
[00:03:48] William: Yeah.
[00:03:48] Frank: And the open rates would tell you who actually read the e-mail.
[00:03:51] William: Yeah.
[00:03:51] Frank: And open rates at Howard for our student e-mails is extremely high, 65%. Now, I have yet to meet a student on this campus that actually tells me they've read the e-mail that we've sent. So, it's like, I don't know who these 65% are because the numbers tell me that it's 65% but they're not actually reading.
[00:04:09] William: Right, right.
[00:04:09] Frank: So, there's more to the story in that space.
[00:04:11] William: Context is important.
[00:04:12] Frank: Yeah. So, you know, would you, would you say that this is also... you know, we talked a little bit in the beginning about a mortgage and appraisals. One of the things that is constantly talked about in terms of difficulty with home ownership in the black community is the fact that appraisals are significantly lower when the appraisers are, you know, of white people that look, go in, and actually, you know, do that work.
[00:04:33] William: Yeah.
[00:04:33] Frank: And I believe that population of black appraisals, or appraisers I should say, are probably lower than 3%-
[00:04:40] William: Yeah.
[00:04:40] Frank: ... inside of that population, too.
[00:04:40] William: Yeah.
[00:04:41] Frank: Would you say that that's another example of, kind of, how the numbers and, and diversity matter of who's interpreting the data?
[00:04:48] William: Absolutely, because keep in mind, data scientists are people and people walk around with their inherent biases. And so, if I'm an appraiser going to a predominantly white neighborhood, nice homes, I will have an inherent bias that these homes, you know, are in a better location, and they're in a better school district, and they may be valued more.
And, and so, so, if that same person goes into a predominantly black neighborhood, there may be questions about, "Well, where are the nearby schools? Are, are the schools really good schools for my client's kids?" And all, and all that. So, so, all that bias comes in. And the reason this is so important is, is because a lot of the bias that winds up in algorithms, they're not necessarily there intentionally. It's almost like an inadvertent inclusion.
The way I look at it sometimes is that in order to build an, an algorithms, there is two components of information that goes in. One aspect goes in by active inclusion. That's the technical specifications. And then the other inclusion is based on passive. And the passive inclusion is, is, is really all those biases that we carry, that we may be building into an algorithm without even knowing it, without even thinking about it.
People are becoming to be sensitive to that now. And because of that, you know, there, there are a lot of sensitivity training, bias training to get people more averse to this. But the best way, I think, is to change the nature of the data science workforce. For example, someone that looks like me will already have an appreciation for, for the black neighborhoods-
[00:06:29] Frank: Okay.
[00:06:29] William: ... that you, you all speak about and, and the importance of, of mortgages and the fairness of, of the mortgages, in fact.
[00:06:37] Frank: Yeah. So, now that, I guess, the traditional sense of what data science is, you know, was a math, kind of, focus, right? It seems like it's, kind of, shifted to be a little bit more social science focused in that space now. Can you talk a little bit about where are the other areas that data science may be applied, like maybe journalism or political science, or what are the other areas outside of just the math of it that this arena really falls into now?
[00:06:59] William: First, let me say that the math and computer science, they remain perennial important components of data science. Those are, are the working of the tools. But let me step back from that and just share with you the way I look at data science. I think about data science this way. Anything that you do that can extract useful information from raw data, that's data science. Okay?
And so, from that perspective, data science can take on the aura of a process and get people to realize that it's not just sequestered or fenced off with a few disciplines, but it impacts a, a wide range of people and activities. For example, journalists typically report a story of what happened and so forth. But now with the complexity of events, then that just was a natural entree for the inclusion of data to help explain the events and help really categorize the impact.
For example, if there's a train development somewhere, a journalist can report that out, but at the same time, inclusion of data can really give more depth of granularity with the impact by talking about, you know, how many people are involved, how many businesses were impacted, how many homes were impacted. And that comes with inclusion of data with the initial story. Now, you can even expand out on, on that.
For example, that's a event in one locale but you can expand out on that and say, now, if you multiply that locale against all the derailments around the country in a certain timeframe, then that would give you a national perspective. And the way certain stories and newspapers are, are now, you can have a map, for example, pinpoint the locations, and the map can actually be interactive, such as if you click on, on a particular location, then that brings to your fingertips all the data associated with that particular location, even the people impacted, and so forth.
And also, when you analyze that data, journalism can also have a predictive impact. For example, by careful analysis of data, journalists can begin to predict where the next event might happen or how severe the next event might be. And that can be important because that can be informative now to decision makers by studying the data from one story and see what went wrong there. Journalists then can make predictions or make recommendations about what the company needs to do to prevent that next time around.
Should the cars be reinforced more to prevent toxic material leaking out, or should it be... What's the ratio then of staff to the number of cars? And all that. So, data has a, has a big role to play in, in journalism. Now, for example, I've also thought about the role of data in politics, political science. And we're in a political season now.
What you're really seeing there is data science in action in real time, because what the pollsters do is really get opinion data from a small group of people and they project that data on what the opinion would be on a large group. They want to know who the front runner is, who the front runner will be, who the front runner should be, and all that kind of stuff.
And on actual election night, the data analysis is even more intense because networks invest a lot of money, time, and resources in being able to predict accurately and rapidly who the winner will be before all the votes are counted. And that's just hardcore data science there. One of the point I make about in the political arena is that for example, policies, the impact of policies on citizens. That is also guided by analysis of data as well.
I mean, for example, if you look at the way police interact with black men, that's really guided by local policies and for the local jurisdictions. And so, you could have data that, that will suggest, well, in this locale, there's been an increase in arrest in the last three years by 20%. Now, if I'm a data scientist, I want to investigate that so I can make recommendation to the decision makers. And depending on my experiential DNA, I will go out and canvass people who I am comfortable with, all right?
[00:11:33] Frank: Mm-hmm.
[00:11:33] William: And so, one data scientist will go out and canvass people, and he would come back with information saying that, "Look, the people in the neighborhood, they feel much safer when they're in their homes. They feel much safer walking down the street, going through the store. So, this policy fairly may be tough, but it is working. It's really serving the people."
Another data scientist can look at the same 20% increase in rate and come away, and would interact with the people that she is comfortable with and say, "During that same period of time when you had the same increase, there's been a 10% increase in the number of black men being shot by police or, or killed by police." And she would come back, back and say, "This policy is causing too much trauma among black families.
So, my recommendation would be that this policy should be modified, discontinued, or replaced." So, you got two data scientists looking at the same data come away with different recommendations, and the recommendation is based on their biases that they carry. And, and those are fairly real circumstances of recommendations that's really fairly current as you, as you know.
[00:12:49] Frank: Yeah. So, and I think that you make such great points, especially because it means that we need more people in the field-
[00:12:52] William: Yeah.
[00:12:52] Frank: ... that look like you and I to be able to get another perspective on what that data could possibly mean.
[00:12:57] William: Yeah.
[00:12:57] Frank: So, you know, universities wanted to attract more black scientists, data scientists I should say, so Howard opened the Center for Applied Science and Analytics. So, what are some of the programs that you have there that are going to help us diversify this field?
[00:13:13] William: First and foremost, that this fall, fall of 2023, we are starting out inaugural class of masters of data science. And so, 30 students have accepted our offer to be part of that class, and we are very excited about that. We are very excited about the program as well because the program has this essential feature combining data science training with social justice. And, and, and that's, like, a staple of our program. And it is all online.
One of the reasons it's online, because they want to take advantage of the broad network of Howard alumni who are all around the nation in the world, make sure they had good access to the program. Another feature of the program that we like is that because we are making a special effort to attract non-STEM students, people from psychology, history, English, because we think they will bring a, a certain freshness and new look to the data science.
And so, what we'd like to see, one of the objectives of the program, is to bring in students from a broad discipline and give them excellent first-class data science training, and hopefully then they will go back to their original disciplines where they will serve as agents of what I call data equity. So, when I say agents of data equity, what am I really saying?
These are individuals who have the training and who have the will and the desire and a position such that they can influence, if not ensure, that data will be implemented equally and fairly across all the segments of society. So, we, we want to produce a cadre of Howard University-produced agents of data equity. And that's one of the, the features of our program.
[00:14:56] Frank: That's great. So, if you are attracting people who may be coming from more of the social sciences areas, the philosophies and others, what if they don't have a strong math skills? How are you supporting, or how's the program supporting people that are, that are transitioning in that space, too?
[00:15:11] William: Yeah, yeah. Now, first of all, we are making a special effort to get non-STEM students. STEM students are welcome as well. We're not, we're not excluding STEM students. What we have done to make the program more accessible to non-STEM students is that we have a designed series of bridge courses that will assume no previous training, no previous background.
And we believe that the time they get through these bridge courses, four courses, then they will be at a place of parity then with STEM students and ready to enter the master's program. So, we think that that's a, a good, a good feature. Now, another aspect also that the master's program I want to mention is that inherent in the program, we have a capstone experience.
The students will take one semester and we will find them positions in their community where they'll be doing data science with companies, agencies, and whether it be fulfilling the data science needs of those organizations that they'd be working with, to get some real-world experience.
[00:16:07] Frank: Yeah, that's great. And, you know, as a professor myself, then I actually teach a capstone course sometimes-
[00:16:11] William: Okay.
[00:16:11] Frank: ... in, in communications and marketing. I think that real life experience is, is so-
[00:16:15] William: Yeah.
[00:16:15] Frank: ... critical to rounding out and making sure those skills have stuck and that they know what they're doing.
[00:16:19] William: Yeah.
[00:16:19] Frank: So, is the master's program the only way that people can engage with the, the data sciences?
[00:16:27] William: No, it's, it's not. I'm also, as, as you mentioned, the principal investigator of Howard University's Research Centers in Minority Institutions program. That's appointed by NIH. And one aspect of that program is something called the Virtual Applied Data Science Training Institute. We call it VADSTI. That's the acronym. What that does, we offer short eight-week workshops in the fundamentals of data science.
We, we offer it to faculty, post-doctoral fellows, researchers. And the idea behind that is to give that cadre of individuals immediate data science skills and know-how that they can incorporate immediately into their research courses. That's been very successful right now. We're in our second iteration of, of that.
And we are also, right now, in the process of requesting funds from NIH for our third iteration. We offer it not only to Howard. This is online. It's virtual. Not only to Howard, but we offer it to HBCUs nationally, and we even had people from other countries sign up for those courses as well.
[00:17:28] Frank: I love that.
[00:17:29] William: Now in the third iteration, we are focusing mostly on undergraduate students because at undergraduate level, students really don't have a lot of exposure to the data science. But we are off the opinion that the data science is everywhere. It affects everybody. So, we want to be proactive in making sure that we equip the Howard undergraduate community and the undergraduate communities, HBCUs around the country to touch some data science exposure.
[00:17:54] Frank: Yeah, I love that. And again, that inclusivity, I think, speaks just to, you know, Howard's nature of-
[00:17:58] William: Yeah.
[00:17:58] Frank: ... of us trying to expand as many places as we can-
[00:18:00] William: Yeah.
[00:18:00] Frank: ... to do that. So, as a data science expert, I have to ask the question, what are the key metrics that you're looking for to know that this program is successful or that the industry is becoming more diverse in ways that, that, that you see fit? And what are some of those metrics you look at?
[00:18:19] William: Well, for the first metric, as far as the program is concerned, we want to see a, a wide array of disciplines coming in. And we want to see people with different interest, different goal, and different expectations that when they leave Howard University program, they will go in different places in society and find their home, their niche.
And we want to see a type of student that really appreciates the uniqueness of the Howard University environment because we, we believe that being associated with the Howard will create a certain sensitivity of fairness and equity across all segments of society. And that's one of the reasons that we wanted to include social justice into this. We want to see, see people embrace the elements of agents of data equity.
Now, this program, the math program, is just starting in the fall, so we have to see over time how this works out and how these individuals are placed and the impact they're having. It's early, right now, for us. But right now, our metrics is, is the kind of people that we are accepting and what they tell us they want to do and how they embrace the Howard philosophy of equity and fairness and data.
[00:19:37] Frank: Love it. Final question, what is your message to those listening to this, our listeners who are maybe interested in either doing one of the programs, the short term or the master's program? Why should they care? You know, what's your final message to them? Why should they care and why should they do this?
[00:19:53] William: Data impacts everybody. People from all disciplines, all backgrounds are impacted by data, but not everyone is aware of that. And if you're not aware of it, then you can't take advantage of it. Okay? So, my message is that realize whether you are aware of it or not.
You are a consumer of data, and if you're not aware of it, then the data you consume is being constructed for you by somebody else. Okay? So, that would be my message. Let me just give you one example. I ask this question sometime. Why do food deserts exist? Okay?
[00:20:37] Frank: That's a good question.
[00:20:39] William: So, food deserts exists because somebody looked at some data and said, "If I put a grocery store in that location, it will fail." Now, if you are aware that your neighborhood is being so affected by data, somebody who’s aware of data, then you can combat that and say, "Right now in this neighborhood, we all buy grocery. But now, we have to travel a long way to get to the grocery that we purchase.
So, we all spend money not only on, on grocery, but getting to and from the grocery store." So, we can harness that data and show if that money was spent locally, then maybe it would support a grocery store. So, that's an example of how understanding and being aware on how data is impacted you.
If you understand that, then you can combat that. If you don't understand it, if you're not, if you're not aware of it, you're not going... it'll impact you because you're just going to say, "Well, there's not a grocery store there, so I got to drive, catch the bus 10 miles to get a carton of milk."
[00:21:41] Frank: Exactly. You heard it from Dr. Southerland. Context is key here. We need more black scientists, data scientists, that are helping to shape the decisions that are made that affect all of our communities. Thank you so much for joining the podcast, Dr. Southerland. This is HU2U, the podcast where we bring today's important topics and stories from Howard University right to you. I am Frank Tramble, today's host, and thank you for listening. HU!
[00:22:06] William: You know!
[00:22:06] Frank: All right. There we go.
[00:22:07] Outro: For more stories from Howard University, visit our award-winning Howard Magazine at magazine.howard.edu, and our award-winning news and information hub, The Dig, at thedig.howard.edu